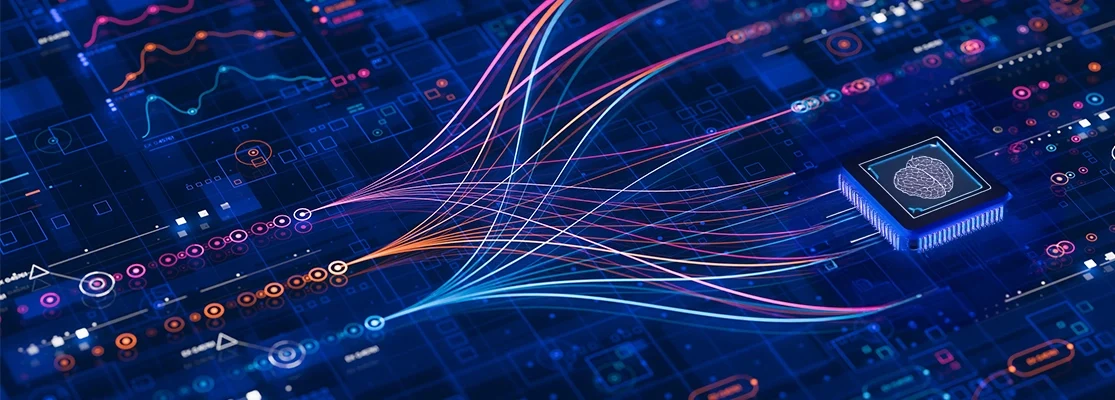
Posted on December 20, 2024 | All
Unpacking AI and ML in Data Analytics: Why It Matters
The digital age has brought an unprecedented explosion of data. From online transactions and customer interactions to sensor readings in industrial equipment, businesses generate vast amounts of data daily. But this deluge presents a paradox—while the opportunities for insights are immense, traditional data analytics methods often falter under the weight of complexity and volume.
Artificial Intelligence (AI) and Machine Learning (ML) are transformative technologies that reshape the way businesses approach advanced data analytics. They offer the speed, scalability, and sophistication needed to turn raw data into actionable insights, empowering organizations to make informed decisions and maintain a competitive edge. AI-powered data analytics revolutionizes businesses by uncovering hidden insights and driving data-driven decisions.
Read more about how AI in data analytics transforms industries by automating complex tasks and providing actionable insights.
The Power of AI and ML in Data Analytics
By incorporating AI in data analytics, businesses can make more informed decisions and gain a competitive edge.
Enhanced Data Processing
Traditional methods struggle with unstructured data, like social media posts, emails, or images. AI algorithms can process these data types efficiently, providing real-time insights. For example, a retail chain can use AI to analyze customer reviews and identify emerging product trends.
Pattern Recognition and Prediction
ML excels at uncovering relationships within data. Consider a logistics company predicting delivery delays by analyzing weather patterns, traffic data, and historical trends, reducing costs and improving customer satisfaction.
Automated Insights Generation
Tasks like data cleaning and feature engineering, once manual and time-intensive, can now be automated. A hospitality business, for instance, can leverage AI to prepare and analyze booking trends, freeing analysts to focus on strategy.
Personalized Customer Experiences
AI enables businesses to cater to individual preferences. For example, an FMCG brand uses AI to create targeted marketing campaigns based on shopping habits, which improves customer engagement and increases sales.
Applications of AI and ML in Data Analytics
AI in data analytics enables organizations to uncover hidden patterns and predict future trends.
This is how:
- Classification: A wine and beverage distributor categorizes customer preferences into wine types, helping tailor marketing.
- Regression Analysis: Predicting peak demand periods in poultry distribution, aiding inventory planning.
- Clustering: Identifying customer clusters for HRMS software to create personalized employee engagement programs.
- Anomaly Detection: Spotting irregularities in manufacturing data to prevent equipment failure.
- Forecasting: Predicting future sales trends for agricultural tools, ensuring stock availability.
Why AI-Driven Analytics Matters
By leveraging real-time analytics with ML, organizations can identify emerging trends, detect anomalies, and respond quickly to market changes.
Faster Time to Insights
AI’s ability to rapidly analyze large datasets allows businesses to respond to real-time market changes. For example, a supply chain manager can adjust operations instantly when forecasts predict disruptions.
Improved Decision Making
AI drives strategic choices by revealing patterns hidden in data. A CFO, for instance, could use AI analytics to predict financial risks, ensuring better investment planning.
Cost Optimization
Automating repetitive analytics tasks saves resources. Retailers, for example, reduce labor costs by automating demand forecasting.
Competitive Advantage
Companies adopting AI analytics gain insights that position them ahead of their competitors. In hospitality, predictive analytics solutions could inform dynamic pricing strategies, maximizing revenue.
Considerations When Implementing AI/ML Analytics
- Data Quality: Poor data quality leads to inaccurate predictions. Businesses must prioritize data cleaning and integrity.
- Explainability: AI models should provide transparent insights to build trust and ensure ethical use. For example, an HR director might want to understand how AI prioritizes employee performance metrics.
RubiCube: Simplifying AI/ML-Driven Analytics
RubiCube seamlessly integrates AI/ML, making analytics accessible and actionable. Whether providing real-time sales insights for retailers or detecting anomalies in manufacturing, RubiCube empowers businesses to turn data into growth opportunities. Real-time analytics with ML allows businesses to make timely decisions by processing and analyzing data as it is generated.
With 50+ visualization options, daily data refreshes, and predictive analytics, RubiCube ensures businesses across industries—retail, HR, logistics, manufacturing, and beyond—can harness the full potential of their data.
CASE STUDY
Decoding QSR Menu Item Performance Using Analytics
A Case Study by CI Global and RubiCube
Executive Summary
This case study explores how analytics can be used to decode Quick-Service Restaurant (QSR) menu item performance. By identifying zero or slow sales items within specific categories and subcategories across stores over a defined time period, QSRs can make informed decisions about menu optimization, including adding new items or removing underperforming ones. This analysis is crucial for understanding how certain menu items affect sales and what alternatives can be considered to enhance revenue.
Goals
- To download and analyze sales data by category
- To enhance menu performance by analysis of sales trends
- To improve data-driven decision-making
Challenges:
1) Lack of visibility into menu item performance:
QSRs often struggle to identify which menu items are driving sales and which are underperforming.
2) Manual data collection and analysis:
Relying on manual methods for data collection and analysis is time-consuming and error-prone.
3) Inconsistent data across stores:
Variations in data collection and reporting practices across different stores can hinder accurate analysis.
4) Difficulty in identifying trends and patterns:
Analyzing large volumes of data manually can be challenging, making it difficult to spot trends and patterns that could inform menu optimization decisions.
5) Limited ability to make data-driven decisions:
Without the right tools and insights, QSRs may struggle to make informed decisions about menu changes.
Solution
Leverage analytics tools in conjunction with Linga POS integration to decode menu item performance and make data-driven decisions for menu optimization. This involved extracting real-time sales data from multiple stores and utilizing RubiCube’s advanced reporting features to identify trends and make data-driven decisions for menu optimization.
Implementation
- Integrate Linga POS: Connect Linga POS to an analytics platform.
- Data collection: Collect real-time sales data from Linga POS.
- Data analysis: Use analytics tools to analyze sales data, identify trends, and compare the performance of different menu items.
- Menu optimization: Make informed decisions about adding, removing, or modifying menu items based on the analysis.
- Regular monitoring: Continuously monitor menu item performance and make adjustments as needed.
Key Features and Benefits
- Real-time data: Access up-to-date information on menu item performance.
- Automated data collection: Reduce manual effort and errors.
- Advanced analytics: Identify trends, patterns, and correlations.
- Data-driven decision-making: Make informed choices about menu optimization.
- Improved profitability: Increase sales and reduce costs through optimized menu offerings.
- Enhanced customer satisfaction: Offer a menu that aligns with customer preferences.
Key Findings:
- Certain menu items positively influence sales.
- Zero or slow sales items may need to be removed or replaced.
- Strategic additions to the menu can drive sales.
Results
- Assisted in identifying sales laggards and deciding on promotions accordingly.
- Helped detect top performers and remove or replace underperforming items.
- Created new menu items based on analysis.
- Improved sales and profitability.
- Enhanced customer satisfaction through optimized menu offerings.
- Increased operational efficiency and customer satisfaction.
Let RubiCube guide your way!
In a world where data is the new currency, leveraging AI and ML for analytics is not optional—it’s essential. Businesses that embrace these technologies are better equipped to navigate challenges, seize opportunities, and achieve sustainable growth. With tools like RubiCube, the journey from raw data to impactful decisions becomes simpler, faster, and more insightful.
Ready to unlock the power of AI/ML-driven analytics?