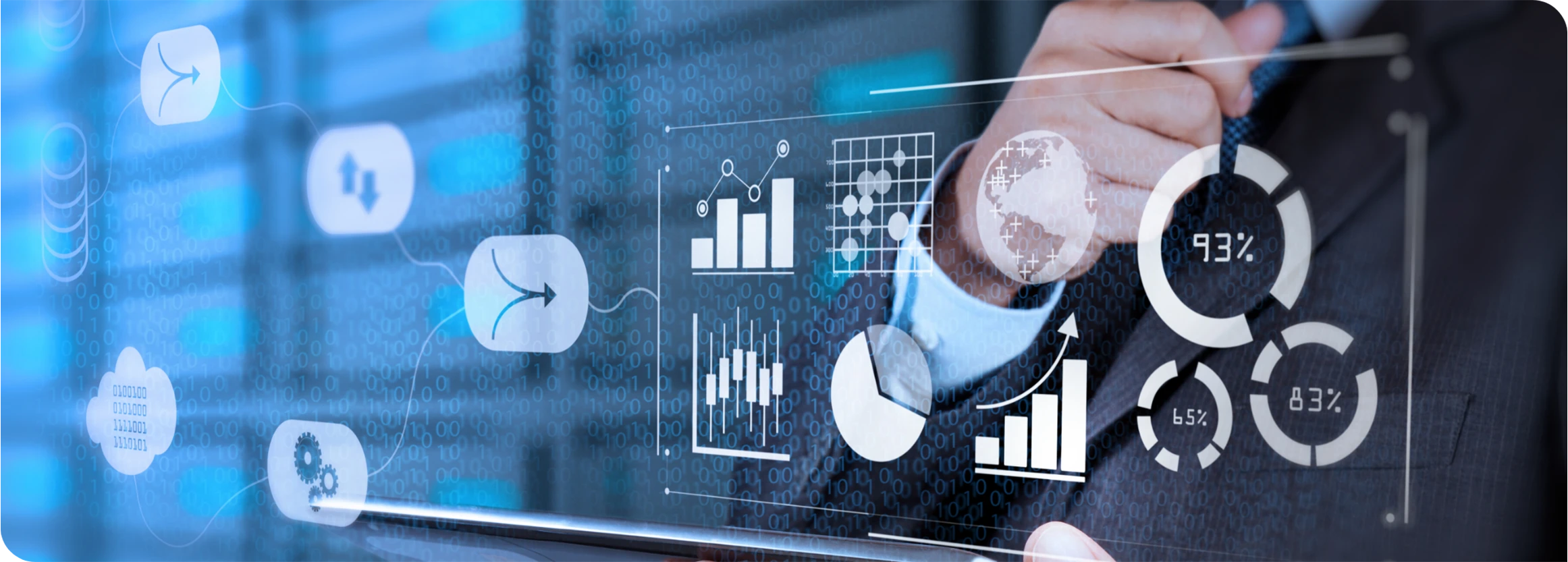
Posted on December 13, 2022 | All
How is predictive analysis the future of Business Intelligence?
Business Intelligence and Data Analytics for ERP
In the age and time of data-dictated rules, business intelligence comprises procedures and infrastructure for collecting, storing, and analyzing the data produced by a company’s systems. It includes data mining, visualization, performance tracking, and descriptive analytics of the plans until that point in time. Predictive analytics is a branch of data analytics that estimates the scope of the future and utilizes data modeling and statistical algorithms to determine future outcomes.
Creating a structure for raw data
Data is scattered and unstructured when derived from the systems because it comes from different sources. By an ETL(Extract, Transform and Load) protocol, you can work on the part of the data that does not affect any part of the running operations. The extraction is when all the desired information is pulled from their respective tables and systems. The transformation includes preparing the collective data for a predictive AI model and generating the desired attributes to fit business needs. The loading step is when the data is ready to be analyzed, and you get insights into the running model of the business.
A journey from statistics to insights
Deriving insights from data is done through data visualization and a thorough analysis of the business situation. Numbers tell the stories that employees hesitate but to derive the pointers, you need to take one extra step. Data insights are achieved through AI/ML models analyzing a dataset. An ML model translates data and numbers into real business value, like targeted advertising efforts and accurate loan predictions. These insights allow users of all skill levels and teams to understand the model’s meaning and where its usefulness could be improved. Through data visualization, see the numbers turn into graphs and pie charts to categorize and visualize the growth of your business.
Predictive Analytics- Vision to the Future
Predictive analytics is the base built in the present for the company’s future. ERP channels the data into data sets that view the present from the perspective of past events. With ERP being used across the industry as the norm, beat your competitors by understanding key areas of improvement and establishing KPIs within your organization. This addition to your ERP structure helps you steer your business away from stagnation points and improve the following parameters:
- Boost client retention and address churn
- Improve core business capabilities
- KYC- Understand your customers better
- Spot opportunities and optimize for productivity
Cleansing Data and Forecasting
Avoid manual processing by investing in specialized software that simplifies the continuous data cleaning that reduces manual processes that are time-consuming and inaccurate. For unstructured and structured data, removing data anomalies and defects helps validate insights and improve the data quality. The broad categories for a forecasting process come under actual and forecast data. The extraction can be automated or through custom-built forecasting tools.
Automation and Machine Learning
ML algorithms are assisting in improving maintenance, repair, and overhaul performance through predictive accuracy broken down into components. Integrating ML intelligence into an ERP allows organizations to get insights into their processes, customers, and workforce simultaneously. In a data-led world, standard ERPs and only live dashboards help in monitoring but don’t give insights into planning. With the help of predictive analytics and ML, invest in the future. With increasing amounts of data, feed data through an AI engine that provides insights and patterns that affect the product life cycle. Automate report-making and essential tasks for rechanneling that time into problem-solving.
Analytical Models
Predictive analysis is not just about predicting; it involves strategizing a method depending on the requirements and where the perspective is coming from. Once you understand the business model you’re following, devising a way to integrate the models is that much easier. The following are some of the most sought-after models:
Forecast Models
A forecast analytical model is based on predictive analytics of how much the demand needs and which way is the right way to invest in a sales team. For example, a forecasting model can help predict consumption and usage with smart meters and give you an accurate forecast of how much power you’ll need to borrow from the powerhouse if you’re a power distributor.
Classification Models
Suppose the likelihood of a business opportunity reciprocating the intentions is high. In that case, it makes better sense to invest money and time in that direction instead of pushing for a broad campaign. A classification model helps categorize customers into yes or no batches; this will help target marketing campaigns well.
Outlier Models
Outlier models spot anomalies like a sudden surge of demand for a particular product in W&B and the need for power in an area or a sudden unexplained dip in receiving input from the power meter of a home. They alert users to data points that stand out from the expected predictions. The model helps track what doesn’t correspond and indicates where the decision-makers and leadership team need to concentrate.
Time Series Models
This analytical model gives insights into changes caused by holidays that come each year, and decoration manufacturers and many food manufacturers get the data on how their stocks need to be to sustain the demand. Turkey during Thanksgiving, plum cake during Christmas, or the market for a particular kind of wine and the expected demand in the future, is one of the best examples for getting data on what you need.
Clustering Models
Clustering is making smaller data groups so companies can categorize an experience unique to this subset. This is one of the patterns used in targeting and segmenting an outreach campaign into different groups. In this analytical model, the best example is identifying what your customer is likely interested in if they’re interested in one product.